audio-ldm
Maintainer: haoheliu

31.798
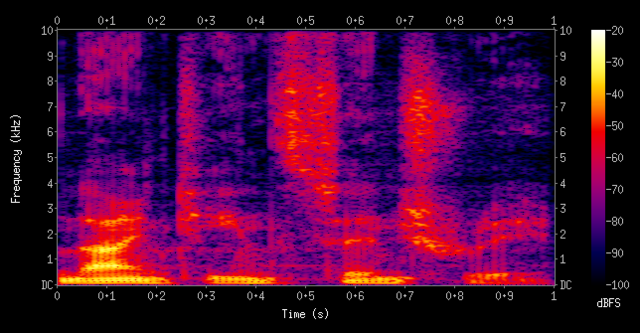
Property | Value |
---|---|
Model Link | View on Replicate |
API Spec | View on Replicate |
Github Link | View on Github |
Paper Link | View on Arxiv |
Text-to-audio generation with latent diffusion models
Get summaries of the top AI models delivered straight to your inbox:
Related Models
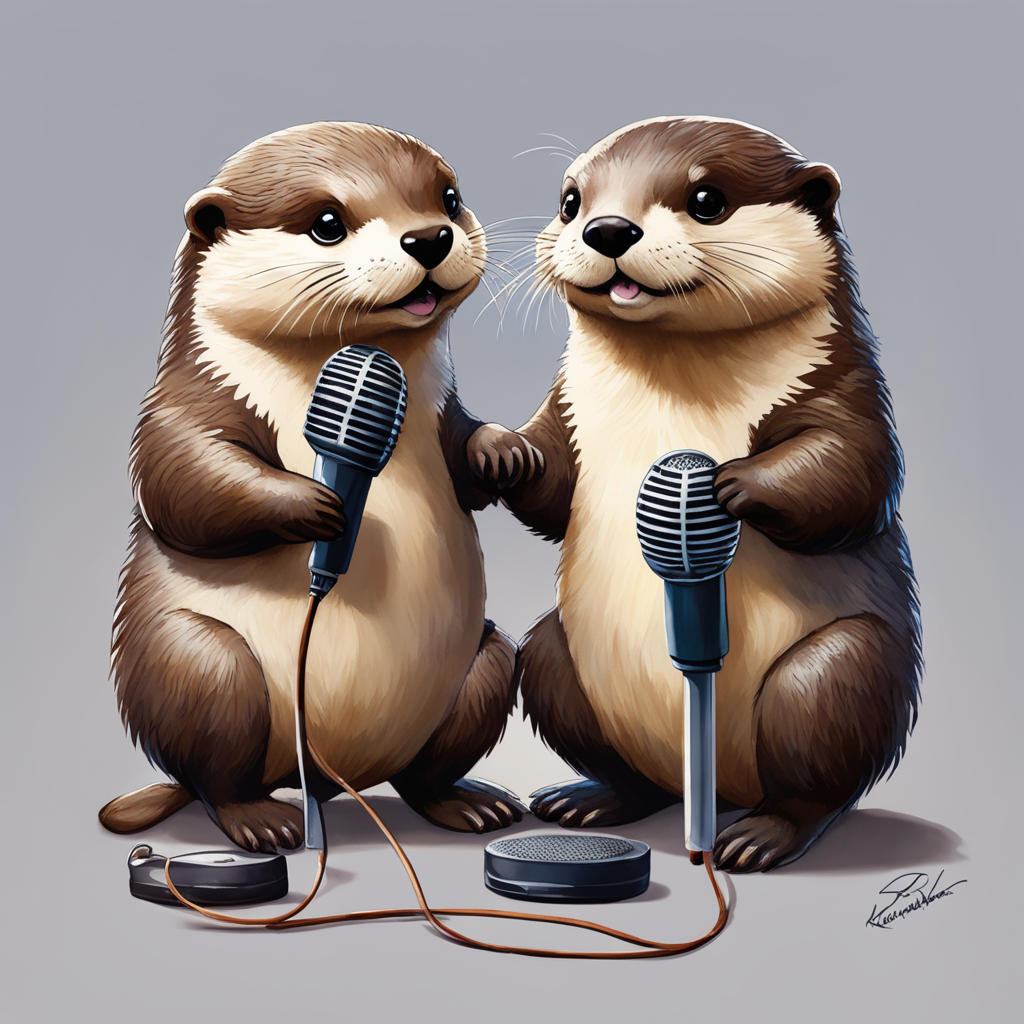
whisper-diarization

205.391
whisper-diarization is a fast audio transcription model that combines the powerful Whisper Large v3 model with speaker diarization from the Pyannote audio library. This model provides accurate transcription with word-level timestamps and the ability to identify different speakers in the audio. Similar models like whisperx and voicecraft also offer advanced speech-to-text capabilities, but whisper-diarization stands out with its speed and ease of use. Model inputs and outputs whisper-diarization takes in audio data in various formats, including a direct file URL, a Base64 encoded audio file, or a local audio file path. Users can also provide a prompt containing relevant vocabulary to improve transcription accuracy. The model outputs a list of speaker segments with start and end times, the detected number of speakers, and the language of the spoken words. Inputs file_string: Base64 encoded audio file file_url: Direct URL to an audio file file: Local audio file path prompt: Vocabulary to improve transcription accuracy group_segments: Option to group short segments from the same speaker num_speakers: Specify the number of speakers (leave empty to autodetect) language: Language of the spoken words (leave empty to autodetect) offset_seconds: Offset in seconds for chunked inputs Outputs segments: List of speaker segments with start/end times, average log probability, and word-level probabilities num_speakers: Number of detected speakers language: Detected language of the spoken words Capabilities whisper-diarization excels at fast and accurate audio transcription, even in noisy or multilingual environments. The model's ability to identify different speakers and provide word-level timestamps makes it a powerful tool for a variety of applications, from meeting recordings to podcast production. What can I use it for? whisper-diarization can be used in many industries and applications that require accurate speech-to-text conversion and speaker identification. Some potential use cases include: Meeting and interview transcription**: Quickly generate transcripts with speaker attribution for remote or in-person meetings, interviews, and conferences. Podcast and audio production**: Streamline the podcast production workflow by automatically generating transcripts and identifying different speakers. Accessibility and subtitling**: Provide accurate, time-stamped captions for videos and audio content to improve accessibility. Market research and customer service**: Analyze audio recordings of customer calls or focus groups to extract insights and improve product or service offerings. Things to try One interesting aspect of whisper-diarization is its ability to handle multiple speakers and provide word-level timestamps. This can be particularly useful for applications that require speaker segmentation, such as conversation analysis or audio captioning. You could experiment with the group_segments and num_speakers parameters to see how they affect the model's performance on different types of audio content. Another area to explore is the use of the prompt parameter to improve transcription accuracy. By providing relevant vocabulary, acronyms, or proper names, you can potentially boost the model's performance on domain-specific content, such as technical jargon or industry-specific terminology.
Updated Invalid Date
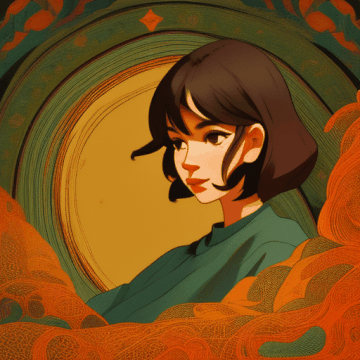
animate-diff

33.808
animate-diff is a plug-and-play module developed by Yuwei Guo, Ceyuan Yang, and others that can turn most community text-to-image diffusion models into animation generators, without the need for additional training. It was presented as a spotlight paper at ICLR 2024. The model builds on previous work like Tune-a-Video and provides several versions that are compatible with Stable Diffusion V1.5 and Stable Diffusion XL. It can be used to animate personalized text-to-image models from the community, such as RealisticVision V5.1 and ToonYou Beta6. Model inputs and outputs animate-diff takes in a text prompt, a base text-to-image model, and various optional parameters to control the animation, such as the number of frames, resolution, camera motions, etc. It outputs an animated video that brings the prompt to life. Inputs Prompt**: The text description of the desired scene or object to animate Base model**: A pre-trained text-to-image diffusion model, such as Stable Diffusion V1.5 or Stable Diffusion XL, potentially with a personalized LoRA model Animation parameters**: Number of frames Resolution Guidance scale Camera movements (pan, zoom, tilt, roll) Outputs Animated video in MP4 or GIF format, with the desired scene or object moving and evolving over time Capabilities animate-diff can take any text-to-image model and turn it into an animation generator, without the need for additional training. This allows users to animate their own personalized models, like those trained with DreamBooth, and explore a wide range of creative possibilities. The model supports various camera movements, such as panning, zooming, tilting, and rolling, which can be controlled through MotionLoRA modules. This gives users fine-grained control over the animation and allows for more dynamic and engaging outputs. What can I use it for? animate-diff can be used for a variety of creative applications, such as: Animating personalized text-to-image models to bring your ideas to life Experimenting with different camera movements and visual styles Generating animated content for social media, videos, or illustrations Exploring the combination of text-to-image and text-to-video capabilities The model's flexibility and ease of use make it a powerful tool for artists, designers, and content creators who want to add dynamic animation to their work. Things to try One interesting aspect of animate-diff is its ability to animate personalized text-to-image models without additional training. Try experimenting with your own DreamBooth models or models from the community, and see how the animation process can enhance and transform your creations. Additionally, explore the different camera movement controls, such as panning, zooming, and rolling, to create more dynamic and cinematic animations. Combine these camera motions with different text prompts and base models to discover unique visual styles and storytelling possibilities.
Updated Invalid Date
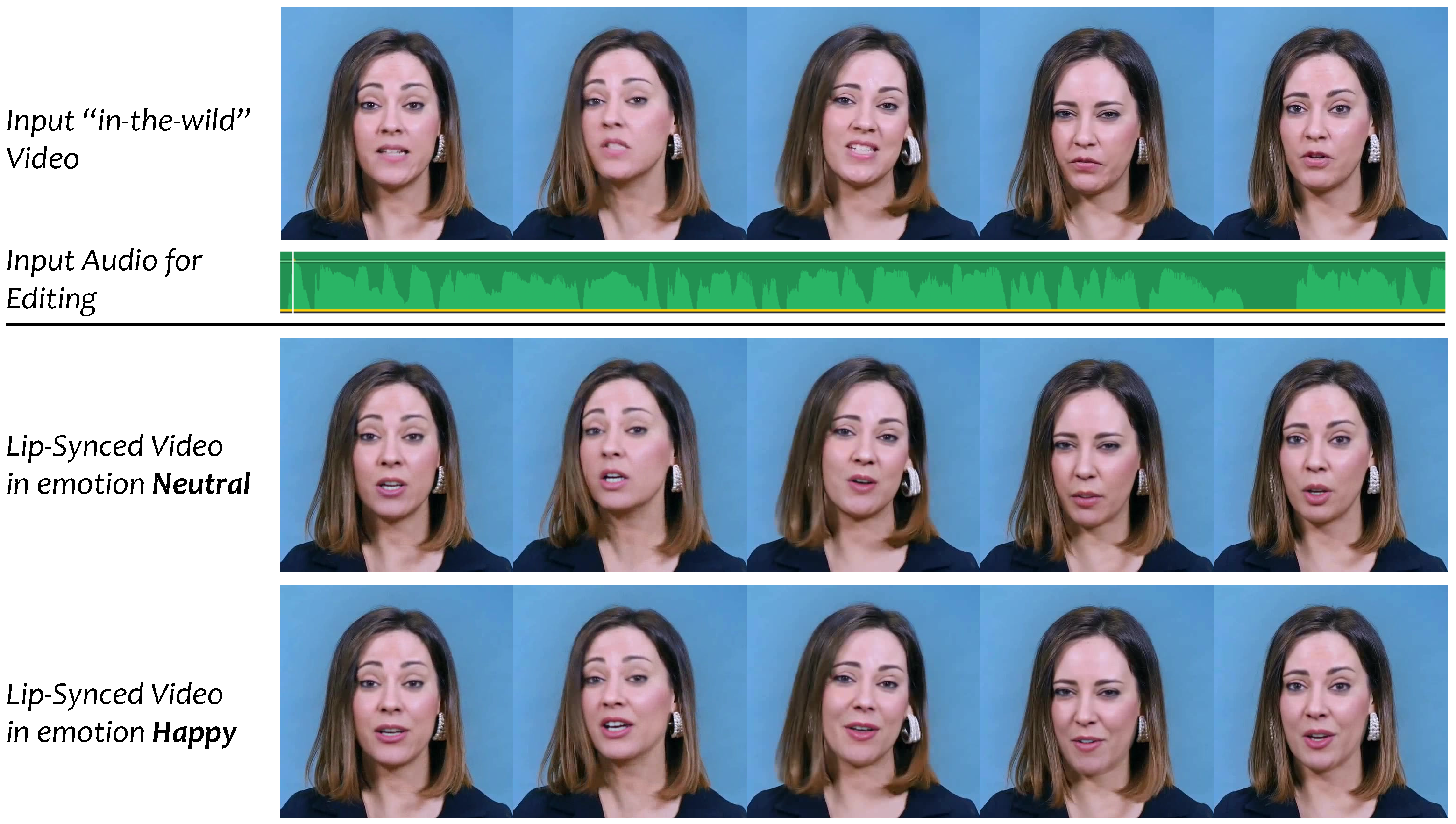
video-retalking

1.614
The video-retalking model is a powerful AI system developed by Tencent AI Lab researchers that can edit the faces of real-world talking head videos to match an input audio track, producing a high-quality and lip-synced output video. This model builds upon previous work in StyleHEAT, CodeTalker, SadTalker, and other related models. The key innovation of video-retalking is its ability to disentangle the task of audio-driven lip synchronization into three sequential steps: (1) face video generation with a canonical expression, (2) audio-driven lip-sync, and (3) face enhancement for improving photo-realism. This modular approach allows the model to handle a wide range of talking head videos "in the wild" without the need for manual alignment or other user intervention. Model inputs and outputs Inputs Face**: An input video file of someone talking Input Audio**: An audio file that will be used to drive the lip-sync Audio Duration**: The maximum duration in seconds of the input audio to use Outputs Output**: A video file with the input face modified to match the input audio, including lip-sync and face enhancement. Capabilities The video-retalking model can seamlessly edit the faces in real-world talking head videos to match new input audio, while preserving the identity and overall appearance of the original subject. This allows for a wide range of applications, from dubbing foreign-language content to animating avatars or CGI characters. Unlike previous models that require careful preprocessing and alignment of the input data, video-retalking can handle a variety of video and audio sources with minimal manual effort. The model's modular design and attention to photo-realism also make it a powerful tool for advanced video editing and post-production tasks. What can I use it for? The video-retalking model opens up new possibilities for creative video editing and content production. Some potential use cases include: Dubbing foreign language films or TV shows Animating CGI characters or virtual avatars with realistic lip-sync Enhancing existing footage with more expressive or engaging facial performances Generating custom video content for advertising, social media, or entertainment As an open-source model from Tencent AI Lab, video-retalking can be integrated into a wide range of video editing and content creation workflows. Creators and developers can leverage its capabilities to produce high-quality, lip-synced video outputs that captivate audiences and push the boundaries of what's possible with AI-powered media. Things to try One interesting aspect of the video-retalking model is its ability to not only synchronize the lips to new audio, but also modify the overall facial expression and emotion. By leveraging additional control parameters, users can experiment with adjusting the upper face expression or using pre-defined templates to alter the character's mood or demeanor. Another intriguing area to explore is the model's robustness to different types of input video and audio. While the readme mentions it can handle "talking head videos in the wild," it would be valuable to test the limits of its performance on more challenging footage, such as low-quality, occluded, or highly expressive source material. Overall, the video-retalking model represents an exciting advancement in AI-powered video editing and synthesis. Its modular design and focus on photo-realism open up new creative possibilities for content creators and developers alike.
Updated Invalid Date
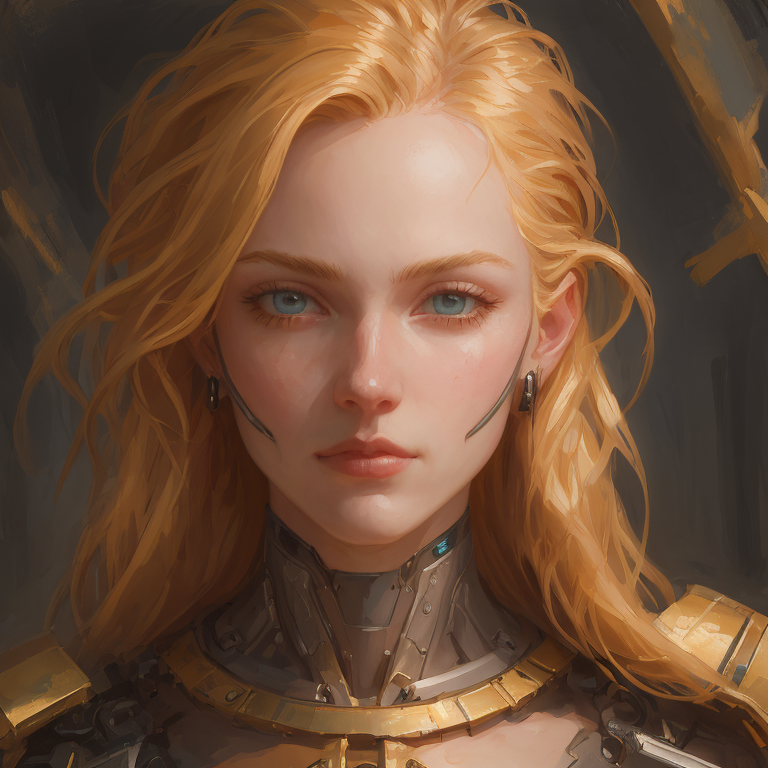
latent-consistency-model

1.1K
The latent-consistency-model is a text-to-image AI model developed by Simian Luo, Yiqin Tan, Longbo Huang, Jian Li, and Hang Zhao. It is designed to synthesize high-resolution images with fast inference, even with just 1-8 denoising steps. Compared to similar models like latent-consistency-model-fofr which can produce images in 0.6 seconds, or ssd-lora-inference which runs inference on SSD-1B LoRAs, the latent-consistency-model focuses on achieving fast inference through its unique latent consistency approach. Model inputs and outputs The latent-consistency-model takes in a text prompt as input and generates high-quality, high-resolution images as output. The model supports a variety of input parameters, including the image size, number of images, guidance scale, and number of inference steps. Inputs Prompt**: The text prompt that describes the desired image. Seed**: The random seed to use for image generation. Width**: The width of the output image. Height**: The height of the output image. Num Images**: The number of images to generate. Guidance Scale**: The scale for classifier-free guidance. Num Inference Steps**: The number of denoising steps, which can be set between 1 and 50 steps. Outputs Images**: The generated images that match the input prompt. Capabilities The latent-consistency-model is capable of generating high-quality, high-resolution images from text prompts in a very short amount of time. By distilling classifier-free guidance into the model's input, it can achieve fast inference while maintaining image quality. The model is particularly impressive in its ability to generate images with just 1-8 denoising steps, making it a powerful tool for real-time or interactive applications. What can I use it for? The latent-consistency-model can be used for a variety of creative and practical applications, such as generating concept art, product visualizations, or personalized artwork. Its fast inference speed and high image quality make it well-suited for use in interactive applications, such as virtual design tools or real-time visualization systems. Additionally, the model's versatility in handling a wide range of prompts and image resolutions makes it a valuable asset for content creators, designers, and developers. Things to try One interesting aspect of the latent-consistency-model is its ability to generate high-quality images with just a few denoising steps. Try experimenting with different values for the num_inference_steps parameter, starting from as low as 1 or 2 steps and gradually increasing to see the impact on image quality and generation time. You can also explore the effects of different guidance_scale values on the generated images, as this parameter can significantly influence the level of detail and faithfulness to the prompt.
Updated Invalid Date